RUDN University mathematician named the main problems of autopilots when detecting pedestrians
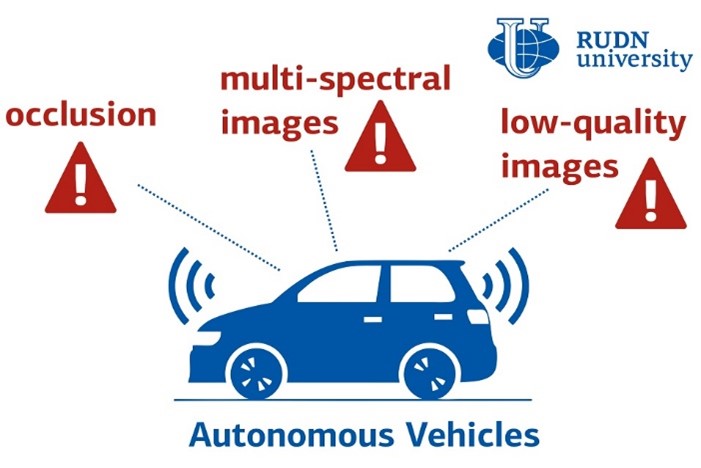
Cars and other self-driving vehicles have already begun to enter everyday life. However, for this technology to be widely adopted, several fundamental characteristics need to be improved. One of them is pedestrian detection. For this, deep learning technologies are used — artificial neural networks. RUDN mathematicians with colleagues from Egypt, Saudi Arabia and China analyzed the latest research in this direction.
“In recent years, autonomous vehicles have become increasingly popular. They improve safety and convenience, reduce fuel consumption, reduce traffic congestion and accidents, save costs and increase reliability. However, before they are fully deployed on the roads, several primary tasks need to be solved. Precise detection of pedestrians is a very difficult task. Deep learning technologies show great potential for solving this problem. We have compiled an overview of the problems of pedestrian detection and the latest advances in solving them using deep learning methods,” Ammar Muthanna, PhD, Junior Researcher at the Research Center for Modeling High-Tech Systems and Infocommunications, RUDN University.
Mathematicians have drawn attention to three main problems that arise when detecting pedestrians using deep learning — obstacles, poor image quality, multispectral images. The latter is a series of images of the same object, but obtained in different ranges. For example, the usual optical image and in the infrared range. It is assumed that the data is obtained from lidars and cameras, from where the deep learning model comes. Mathematicians have reviewed the different approaches that are used to build such models, and called their advantages and disadvantages.
RUDN mathematicians noticed that different construction methods give not only different results, but also different time and accuracy of work. For example, more modern methods give a more accurate answer, but it takes more time. Therefore, the scientists concluded, it is important to implement an approach that can find a balance between accuracy and speed. And one that will meet practical goals. Mathematicians also noticed that the data available for training is not heterogeneous enough and may not give a complete picture. For example, images in the optical range work better during the day, and in the infrared — in the dark. For the algorithm to be efficient around the clock, large heterogeneous data sets are needed for training.
Among the goals for future research in this area, RUDN mathematicians named the detection of small and obstructed objects, work in poor lighting, a combination of 3 D and 2 D approaches. The researchers also suggest focusing on improving speed and accuracy.
Results published in Electronics.
Matilda Pavlovna Mityaeva was born in 1925. In November 1942, she volunteered for frontline duty. She participated in the Great Patriotic War from November 1942 to June 1945 as part of the 53rd Infantry Division of the 475th Infantry Regiment. She was wounded twice.
The team led by Sergey Zyryanov, Head of the Department of General and Clinical Pharmacology, became the winner of the All-Russian competition of scientific projects "Technologies for Human Health".
RUDN University constantly adapts to the changes of the modern world and responds to challenges flexibly. This allows us to keep the standard of a world-class research university. The sphere of science is no exception. Peter Dokukin, Head of the Research Division, presented the updated R&D Programme at the meeting of the RUDN University Academic Council.
Matilda Pavlovna Mityaeva was born in 1925. In November 1942, she volunteered for frontline duty. She participated in the Great Patriotic War from November 1942 to June 1945 as part of the 53rd Infantry Division of the 475th Infantry Regiment. She was wounded twice.
The team led by Sergey Zyryanov, Head of the Department of General and Clinical Pharmacology, became the winner of the All-Russian competition of scientific projects "Technologies for Human Health".
RUDN University constantly adapts to the changes of the modern world and responds to challenges flexibly. This allows us to keep the standard of a world-class research university. The sphere of science is no exception. Peter Dokukin, Head of the Research Division, presented the updated R&D Programme at the meeting of the RUDN University Academic Council.