RUDN mathematician improved the machine learning algorithm for recognizing images from satellites
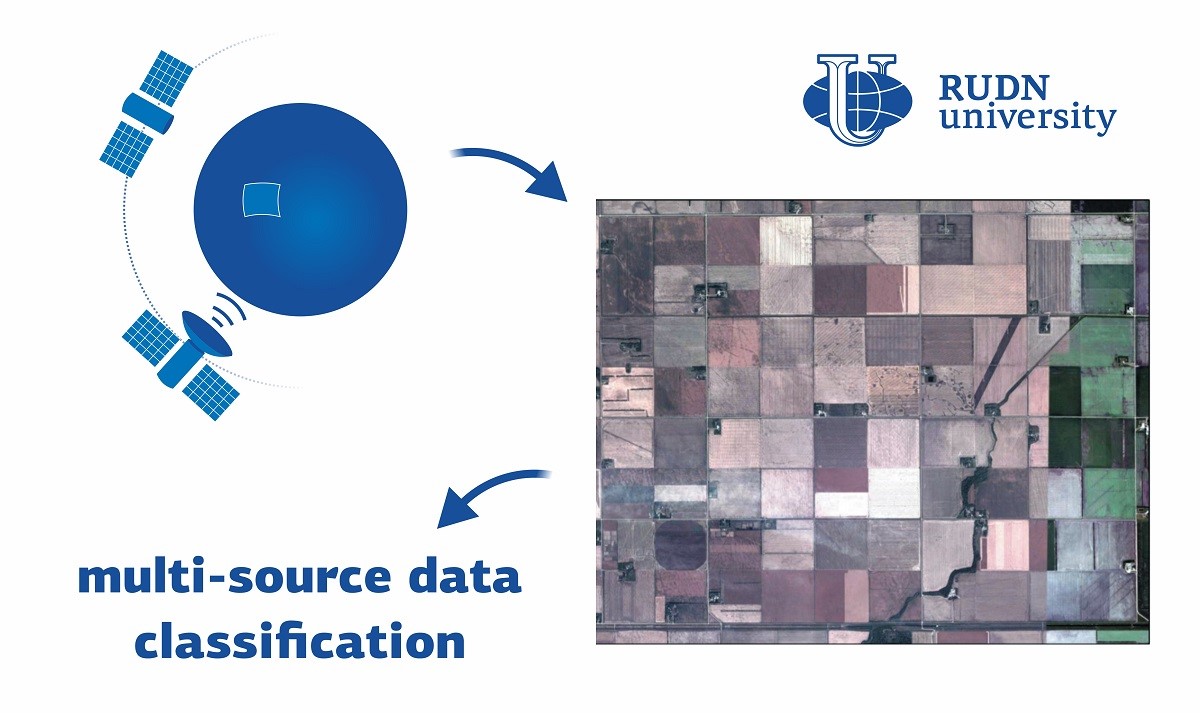
Modern satellite and radar systems can be used to automatically monitor earthquakes, volcanic eruptions, fires, and other disasters, as well as to monitor the condition of soil, vegetation, and rivers. To automate this process, complex algorithms need to recognize and classify objects, allowing the computer to understand from a set of pixels what is depicted on the image. Machine learning is used for this purpose, a computer “looks through” thousands of examples and thus learns to recognize images independently. To improve machine learning results, a combination of several training algorithms is often used. This yields more accurate solutions than each of them separately. The RUDN mathematician developed such an ensemble method using three algorithms to process data from multiple sources.
Mathematicians used data from five RapidEye mini-satellites and the UAVSAR airborne radar on July 5 and 7, 2012, they captured the same area in Canada. The RapidEye imagery was acquired in five bands of the light spectrum: blue (B), green (G), red ®, near-infrared (NIR) and a region called “red edge” (RE) where the reflection of green vegetation is dramatically enhanced. The data contained 38 features: spectral channels, vegetation indices, texture parameters, and etc. Their spatial resolution, that is, the minimum object size distinguishable in the images was about five meters. UAVSAR radar images included 49 different features, with a spatial resolution of about 15 meters. Mathematicians compared the images with reference data on the area collected in the summer of 2012. They identified seven types of plants, broadleaf plants, rapeseed, corn, oats, peas, soybeans and wheat. The new algorithm was “trained” based on examples of images and planting type data, and then compared its prediction with the results of other programs based on a similar principle.
The new method showed higher accuracy in interpreting images, both on large and limited amounts of examples for training algorithms. If training was performed on 5% of all data, the new algorithm recognized images correctly at least 65% of the time, while the other algorithms were correct in 52-60% of cases. With an increase in the share of training data to 50% of the total volume, the accuracy of the new algorithm increased to almost 90%, and the other algorithms increased to 75-86%. Thus, the application of the new algorithm was found to be more effective.
“Our method can be proposed for a land use and land cover classification system using data from different sources. For example, Landsat or Sentinel constellation satellites,” Vladimir Razumny, Ph.D., Associate Professor at the RUDN Mechanical and Mechatronics Engineering department.
The article was published in International Journal of Image and Data Fusion
Matilda Pavlovna Mityaeva was born in 1925. In November 1942, she volunteered for frontline duty. She participated in the Great Patriotic War from November 1942 to June 1945 as part of the 53rd Infantry Division of the 475th Infantry Regiment. She was wounded twice.
The team led by Sergey Zyryanov, Head of the Department of General and Clinical Pharmacology, became the winner of the All-Russian competition of scientific projects "Technologies for Human Health".
RUDN University constantly adapts to the changes of the modern world and responds to challenges flexibly. This allows us to keep the standard of a world-class research university. The sphere of science is no exception. Peter Dokukin, Head of the Research Division, presented the updated R&D Programme at the meeting of the RUDN University Academic Council.
Matilda Pavlovna Mityaeva was born in 1925. In November 1942, she volunteered for frontline duty. She participated in the Great Patriotic War from November 1942 to June 1945 as part of the 53rd Infantry Division of the 475th Infantry Regiment. She was wounded twice.
The team led by Sergey Zyryanov, Head of the Department of General and Clinical Pharmacology, became the winner of the All-Russian competition of scientific projects "Technologies for Human Health".
RUDN University constantly adapts to the changes of the modern world and responds to challenges flexibly. This allows us to keep the standard of a world-class research university. The sphere of science is no exception. Peter Dokukin, Head of the Research Division, presented the updated R&D Programme at the meeting of the RUDN University Academic Council.